In today's rapidly advancing world of AI technology, ensuring ethical compliance is more crucial than ever. As we integrate artificial intelligence into our daily lives, understanding the ethical implications and striving for responsible innovation should be at the forefront of our conversations. From data privacy to algorithmic fairness, these topics demand our attention and thoughtful consideration. Join us as we explore the essential elements of AI ethical compliance and why they matter for a sustainable future.
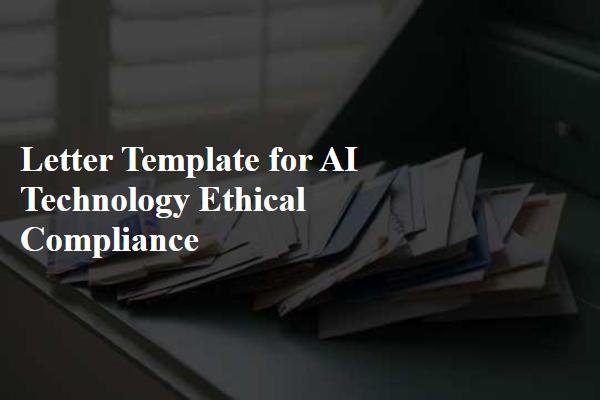
Privacy and data protection
AI technology compliance with ethical standards prioritizes privacy and data protection for users. Data collection practices must adhere to regulations like the General Data Protection Regulation (GDPR) in Europe, which imposes strict guidelines on the processing of personal information. Important measures include clear consent protocols for data usage, secure storage methods to protect sensitive information, and the implementation of privacy by design principles from the outset. Organizations must conduct regular audits to ensure compliance and transparency in data handling. User rights, such as data access and the right to be forgotten, must be actively upheld to foster trust and accountability in AI systems.
Accountability and transparency
AI technology requires strict accountability and transparency to ensure ethical compliance in its deployment and use. Organizations developing AI systems must adhere to data protection regulations (such as GDPR in Europe) to safeguard personal information. Implementing audit trails enables tracking decisions made by algorithms, providing a clear accountability framework for stakeholders. Additionally, fostering transparency involves creating understandable documentation outlining how AI models function, including the data used for training and decision-making processes. Engaging with external auditors can enhance trust in AI solutions by validating ethical adherence. Moreover, collaboration with diverse stakeholder groups, including ethicists and community representatives, can improve the ethical landscape by addressing potential biases and societal impacts effectively.
Fairness and non-discrimination
AI systems must prioritize fairness and non-discrimination to ensure equitable outcomes across diverse demographics. Algorithms designed for predictive analytics can inadvertently perpetuate biases if trained on skewed datasets, resulting in discriminatory practices against specific groups based on race, gender, or socioeconomic status. Implementing audit strategies such as algorithmic fairness assessments is crucial, utilizing metrics like disparate impact and equal opportunity for evaluation. Regular updates and retraining on representative datasets will enhance the AI's adaptability, fostering inclusivity and reducing systemic bias. Organizations should establish transparency through clear reporting on AI decision-making processes to build trust among users and stakeholders.
Safety and security measures
Ethical compliance in AI technology emphasizes the importance of safety and security measures in mitigating potential risks. Implementing robust safety protocols for artificial intelligence systems, including the use of advanced encryption methods, is essential to prevent unauthorized access to sensitive data. Regular audits and assessments of AI algorithms ensure transparency and accountability, addressing biases present in training datasets that could skew decision-making processes. Developing clear guidelines, such as those advocated by organizations like IEEE and ISO, establishes benchmarks for ethical AI deployment. Maintenance of user privacy, adherence to legal regulations (such as GDPR for data protection in the European Union), and ongoing education about ethical uses of AI contribute to a secure technological landscape. Engaging in interdisciplinary collaborations with ethicists, technologists, and legal experts fosters a comprehensive approach to AI safety.
Regulatory and legal adherence
AI technologies must adhere to rigorous ethical compliance standards, ensuring regulatory and legal alignment with frameworks such as the General Data Protection Regulation (GDPR) in Europe and the California Consumer Privacy Act (CCPA) in the United States. These laws mandate the protection of personal data, emphasizing the necessity for transparent data processing practices, user consent, and stringent data security measures. Organizations utilizing AI, particularly in sectors like healthcare and finance, must implement robust risk assessment procedures to identify potential biases in algorithms, which could lead to discriminatory outcomes. Compliance with these regulations not only mitigates legal risks but also fosters trust among users and stakeholders, promoting a responsible AI ecosystem. Regular audits and adherence to ethical guidelines set by institutions such as the IEEE (Institute of Electrical and Electronics Engineers) further support legal compliance, enhancing accountability within AI development processes.
Comments