Are you gearing up to submit your manuscript and want to ensure your data is rock-solid? ItÂ’s crucial to verify that your data integrity meets the highest standards before sharing your research with the world. In this article, weÂ’ll walk you through essential steps for conducting a thorough data integrity check, helping you avoid common pitfalls and elevating the quality of your work. So, letÂ’s dive in and empower your manuscript with impeccable data reliability!
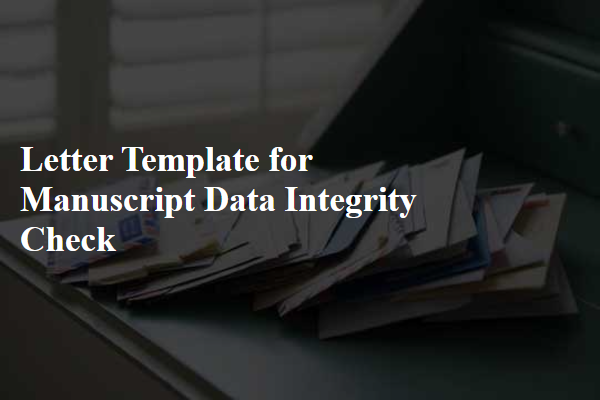
Clarity in Data Presentation
Clarity in data presentation is crucial for ensuring the integrity of research findings within scientific manuscripts. Structured formats, such as tables and figures, can significantly enhance understanding by effectively summarizing complex datasets. Adherence to standardization in visual components is essential, as guidelines from organizations like the American Psychological Association (APA) or the Council of Science Editors (CSE) serve to maintain consistency across disciplines. Furthermore, providing clear captions and legends alongside visual data representation helps contextualize findings, allowing readers to quickly grasp significant trends or anomalies. Rigorous data verification processes should also be employed, with checks against common errors such as mislabeling or incorrect scaling. Attention to these details not only bolsters the manuscript's credibility but also fosters trust among the research community and stakeholders.
Consistency of Data Metrics
A manuscript data integrity check is crucial for ensuring the consistency of data metrics in scientific research. Consistency refers to the reliability and accuracy of collected data, vital for drawing valid conclusions. In the context of clinical studies, for example, discrepancies in patient demographic metrics (age, gender representation) can impact study outcomes significantly. Rigorous checks, including statistical analysis and verification against original data sources, serve as essential steps to confirm that metrics align with established protocols and standards. Moreover, implementing software tools, such as R or Python scripts, can automate the monitoring process, identifying anomalies that could compromise data validity. Researchers must document these checks meticulously in their methods section to uphold transparency and reproducibility, fundamental principles in scientific inquiry.
Verification of Data Sources
Verification of data sources is crucial for ensuring the integrity of research findings in academic manuscripts. Accurate identification of primary data (original collected data) and secondary data (previously published data) must occur during the research process. Scrutiny includes evaluating dataset sources (such as government databases, scientific repositories), assessing data collection methods (surveys, experiments), and reviewing compliance with ethical standards (Institutional Review Boards approval). Additionally, cross-referencing cited studies (using tools like CrossRef or Google Scholar) checks for reliability, verifying authorship, and confirming publication status (peer-reviewed, retracted). Maintaining a rigorous data verification process at these stages guarantees the reliability and validity of the conclusions drawn from the research.
Compliance with Ethical Standards
Ensuring data integrity in research manuscripts is paramount for maintaining compliance with ethical standards. This involves implementing rigorous methods for data collection, analysis, and reporting. For example, using statistical software like SPSS or R ensures accurate data analysis, while transparent methodologies enable reproducibility and verification of results. Ethical considerations also include obtaining informed consent from participants, following guidelines set by institutional review boards (IRBs), and maintaining confidentiality. Moreover, adherence to guidelines such as the Declaration of Helsinki emphasizes the importance of ethical conduct in human subject research. Continuous training for researchers on ethical standards and data management fosters a culture of integrity and accountability within academic communities.
Documentation of Data Alterations
Data integrity checks are crucial for maintaining the reliability of research findings. Documentation of data alterations must include specific details such as the date of modification, the reason for the change, and the individual responsible for the revision. For instance, in clinical trials, changes to patient data (e.g., demographic information or treatment outcomes) should be logged meticulously to ensure traceability. Utilizing software tools like version control systems can further enhance the accountability of data management processes. Maintaining a comprehensive audit trail ensures compliance with regulatory standards set by institutions like the FDA or EMA and fosters confidence in the integrity of the research outcomes.
Comments